MedComm-Future Medicine | Accelerating the integration of ChatGPT and other large-scale AI models into biomedical research and healthcare

Open the phone and scan
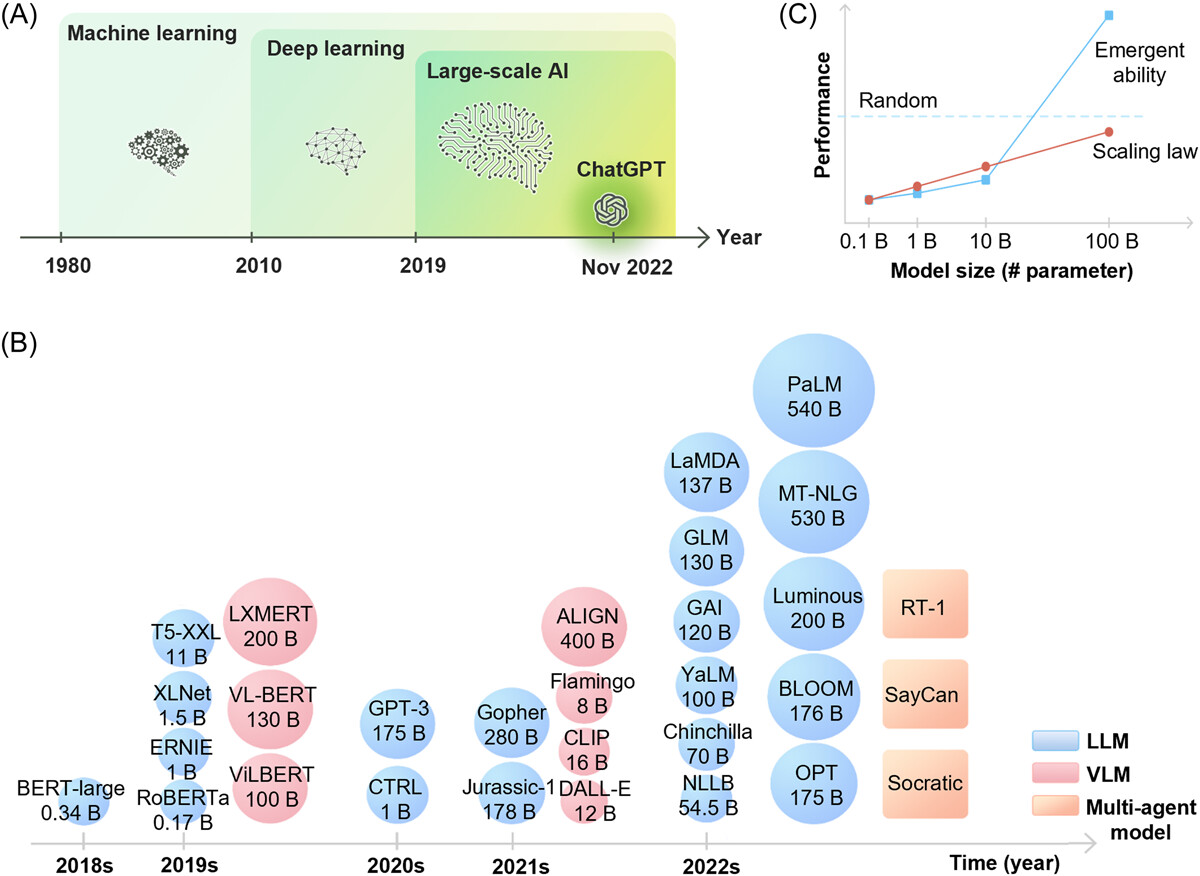
Large-scale AI models. (A) Comparison of large-scale AIs with deep learning and machine learning. (B) Overview of the most recent advanced large-scale AI models and their sizes. A summary of the latest advanced large-scale AI models, including Large Language Models (represented in blue), Vision-Language Models (represented in red), and Multiagent Models (represented in orange). The size of each model is also depicted. (C) The impact of model size on performance. Red Line: The performance of the model increases linearly as the model size increases exponentially in accordance with the Scaling Law. Blue Line: Some large-scale AI models display emergent abilities as their size increases. Initially, their performance on a task is randomly distributed (as indicated by the dashed blue line). However, as the model grows larger, it reaches a threshold where its performance suddenly improves, demonstrating emergent abilities.
Large-scale artificial intelligence (AI) models such as ChatGPT have the potential to improve performance on many benchmarks and real-world tasks. However, it is difficult to develop and maintain these models because of their complexity and resource requirements. As a result, they are still inaccessible to healthcare industries and clinicians. This situation might soon be changed because of advancements in graphics processing unit (GPU) programming and parallel computing. More importantly, leveraging existing large-scale AIs such as GPT-4 and Med-PaLM and integrating them into multiagent models (e.g., Visual-ChatGPT) will facilitate real-world implementations. This review aims to raise awareness of the potential applications of these models in healthcare. We provide a general overview of several advanced large-scale AI models, including language models, vision-language models, graph learning models, language-conditioned multiagent models, and multimodal embodied models. We discuss their potential medical applications in addition to the challenges and future directions. Importantly, we stress the need to align these models with human values and goals, such as using reinforcement learning from human feedback, to ensure that they provide accurate and personalized insights that support human decision-making and improve healthcare outcomes.
Article Access: https://doi.org/10.1002/mef2.43
More about MedComm-Future Medicine: https://onlinelibrary.wiley.com/journal/27696456
Looking forward to your contributions.
Review : Chunhua Li